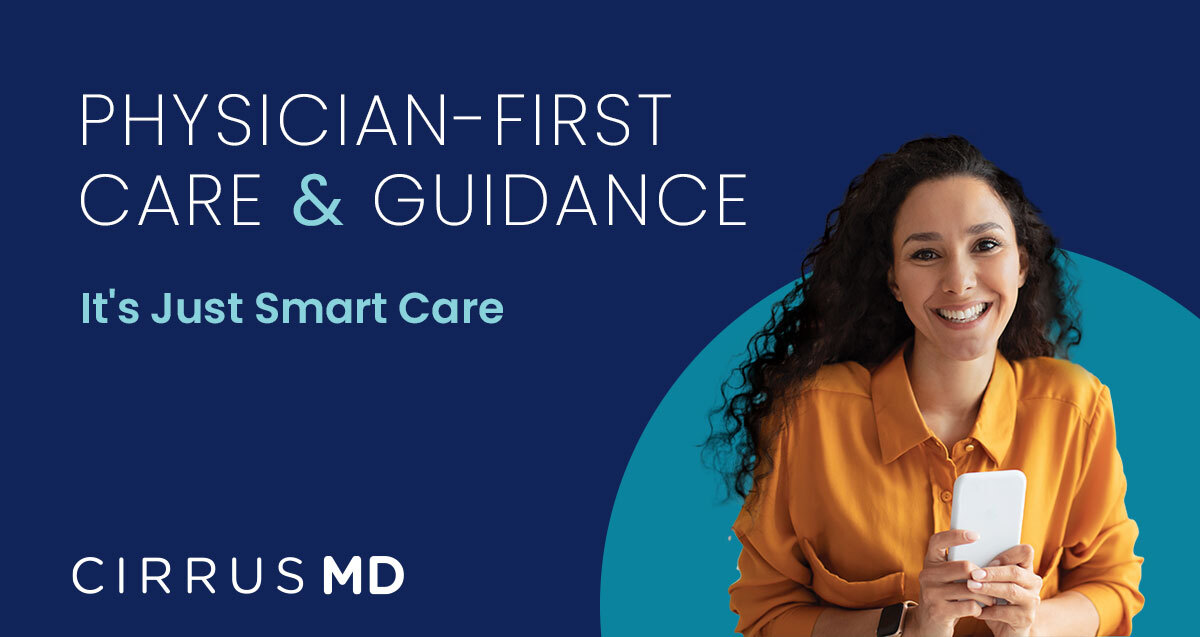
Introduction
There has been an explosion of interest – and concern – around the use of artificial intelligence (AI) and machine learning (ML) in healthcare delivery. The safety and effectiveness of leveraging these technologies really come down to the specific use case.
Patients want the assurance and trust that comes with a qualified and experienced physician directing their care – not a chatbot. For example, a recent Pew Research survey of U.S. adults found about eight-in-ten (79%) would not want to use an AI chatbot if they were seeking mental health support.
Physicians wanting to use their knowledge, skills and experience in care delivery don’t want to contend with technology coming between them and their patients. However, in a recent Medscape survey of U.S. physicians, over half (56%) described some level of enthusiasm about AI offering diagnosis and treatment options to supplement their recommendations.
“Clinical decisions influenced by AI must be made with specified human intervention points during the decision-making process.” - American Medical Association
Instead of developing AI/ML applications that take the place of physician/patient interactions or create barriers between the parties, the most advantageous approach is to employ these technologies to support physicians during episodes of care. Positioning the power of AI/ML to analyze vast volumes of data and surface insights behind the physician while they are facing the patient preserves the value of the physician/patient personal connection.
This paper explores use of AI/ML in a virtual environment using a physician-first care and guidance model to empower physicians with real-time information at their fingertips during an episode of care. Improving efficiency in care interactions by surfacing valuable insights to the physician for their evaluation and potential use – as opposed to them having to search for information – supports the delivery of higher quality care to a greater number of patients at a lower cost.
The promise of AI and ML in healthcare delivery
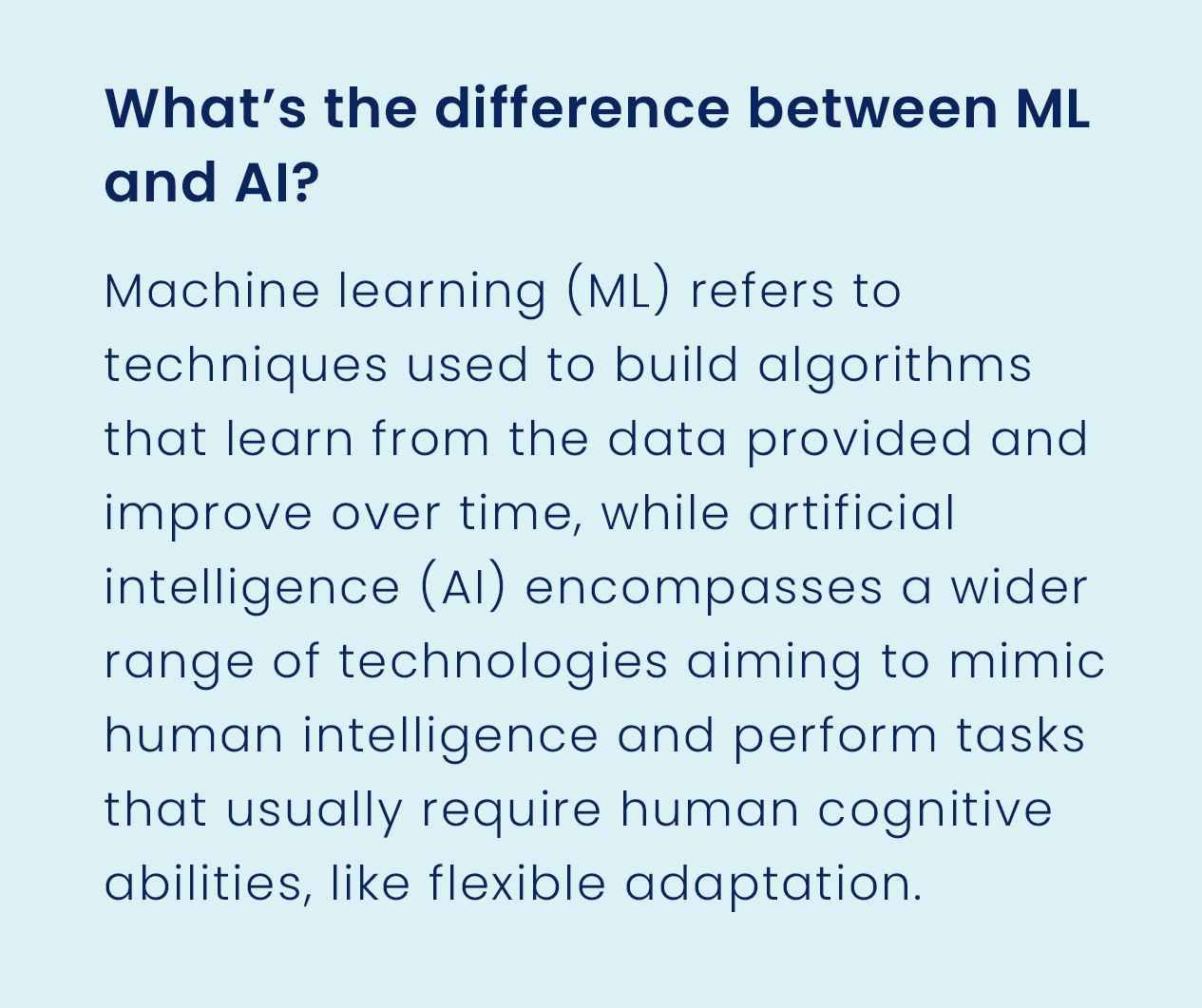
One in four Americans do not have a relationship with a primary care physician (PCP), with the U.S. experiencing a shortage of anywhere between 17,000 to 52,000 PCPs.4 These shortages are driving patients to more expensive care settings (e.g., emergency departments, urgent care centers) and/or restricting them from undergoing routine care and tests.
Challenges to PCP retention include “limited flexibility in schedules, time spent coordinating patient care, and dissatisfaction with the increased burden of non-medical tasks (form completion, pre-authorizations for medications, and similar tasks) that are not reimbursed in the fee-forservice model.” Improving efficiency in care interactions can help alleviate the PCP shortage burden by providing more time for direct patient care activities.
The application of AI and ML models, with their ability to analyze tremendous volumes of data and surface information and insights, holds significant potential to improve efficiency in healthcare delivery and patient outcomes. As the authors of a study on ML in healthcare published in the International Journal of Intelligent Networks stated:
“Using ML for healthcare can open up a world of possibilities in this field. It frees up healthcare providers’ time to focus on patient care rather than searching or entering information.”
In a 2023 American Medical Association (AMA) survey of over 1,000 physicians on their sentiments toward the use of AI in healthcare, 56% of respondents indicated that AI can best help with administrative burdens through automation.
“The utilization of AI and ML in healthcare has the potential to redefine diagnostic accuracy, treatment personalization, and overall healthcare system efficiency. As these technologies continue to evolve, their impact on clinical decision-making and patient care is becoming more profound.”
Risks of AI and ML integrations in healthcare
While AI and ML hold potential to support clinical care delivery, some applications to date, most notably those that have attempted to put the technology in the place of a physician or between the physician and patient, have revealed the dangers of this approach.
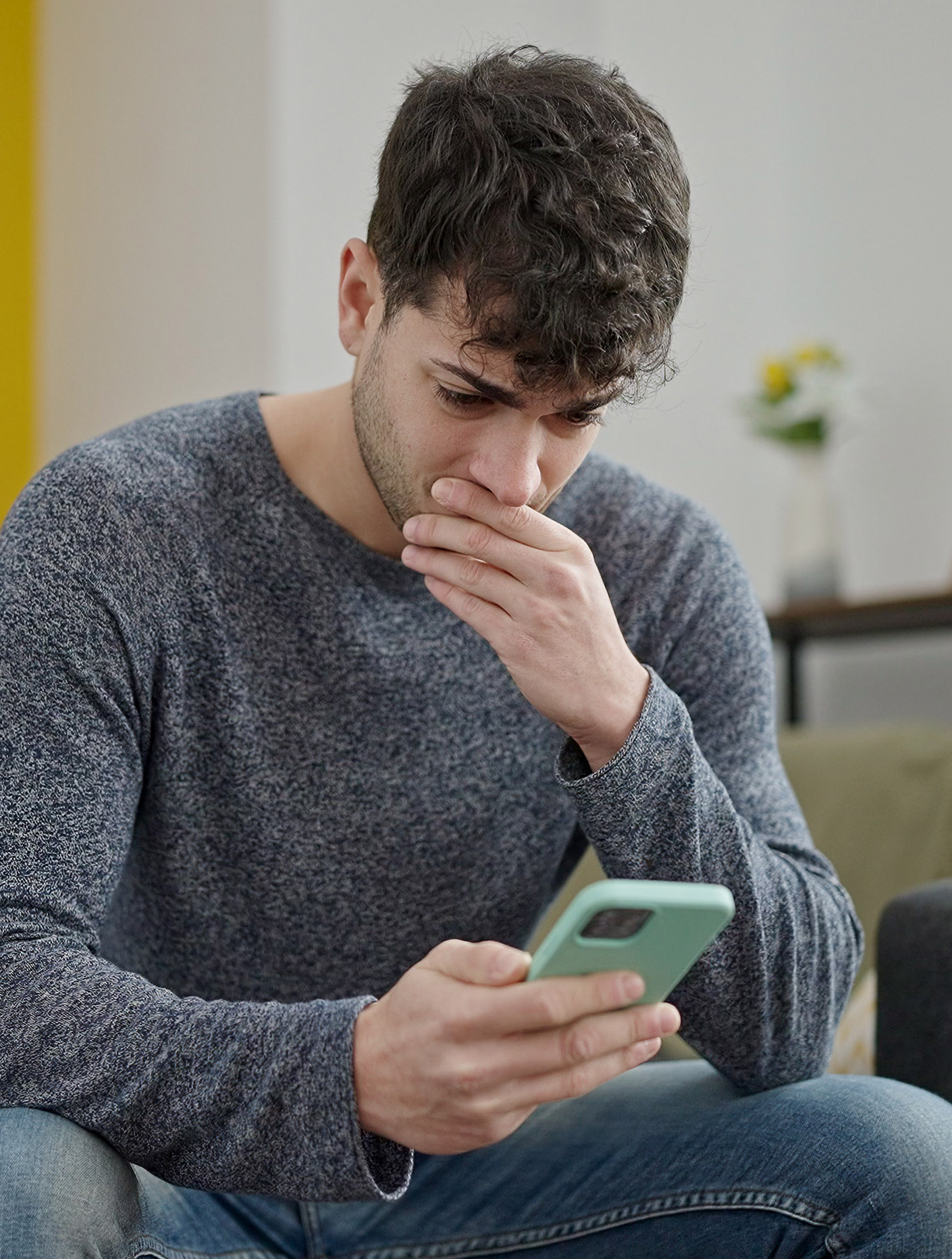
Conflicting advice to cancer patients
“Although AI chatbots can gather cancer information from reputable sources, their responses can include errors, omissions, and language written for health care professionals rather than for patients,” stated researchers from Mass General Brigham, Harvard Medical School, Boston Children’s Hospital, and Memorial Sloan Kettering Cancer Center. They published their study findings in JAMA Oncology on August 24, 2023.
The researchers evaluated the LLM chatbot’s performance on providing breast, prostate, and lung cancer treatment recommendations in accordance with National Comprehensive Cancer Network (NCCN) guidelines. They found one-third of the chatbot’s recommended treatments were at least partially misaligned with NCCN guidelines, with recommendations varying based on how a question was posed.
Perhaps most alarming, “the chatbot was most likely to mix in incorrect recommendations among correct ones, an error difficult even for experts to detect.” They concluded, “Clinicians should advise patients that LLM chatbots are not a reliable source of treatment information.”
“When (AI) sounds so human and confident, it can be hard to distinguish between what is accurate and what is not,” cautions Yale Medicine nephrologist F. Perry Wilson, MD. “In the end, trust your doctors, as we are the ones who have the responsibility to look out for your best interest.”
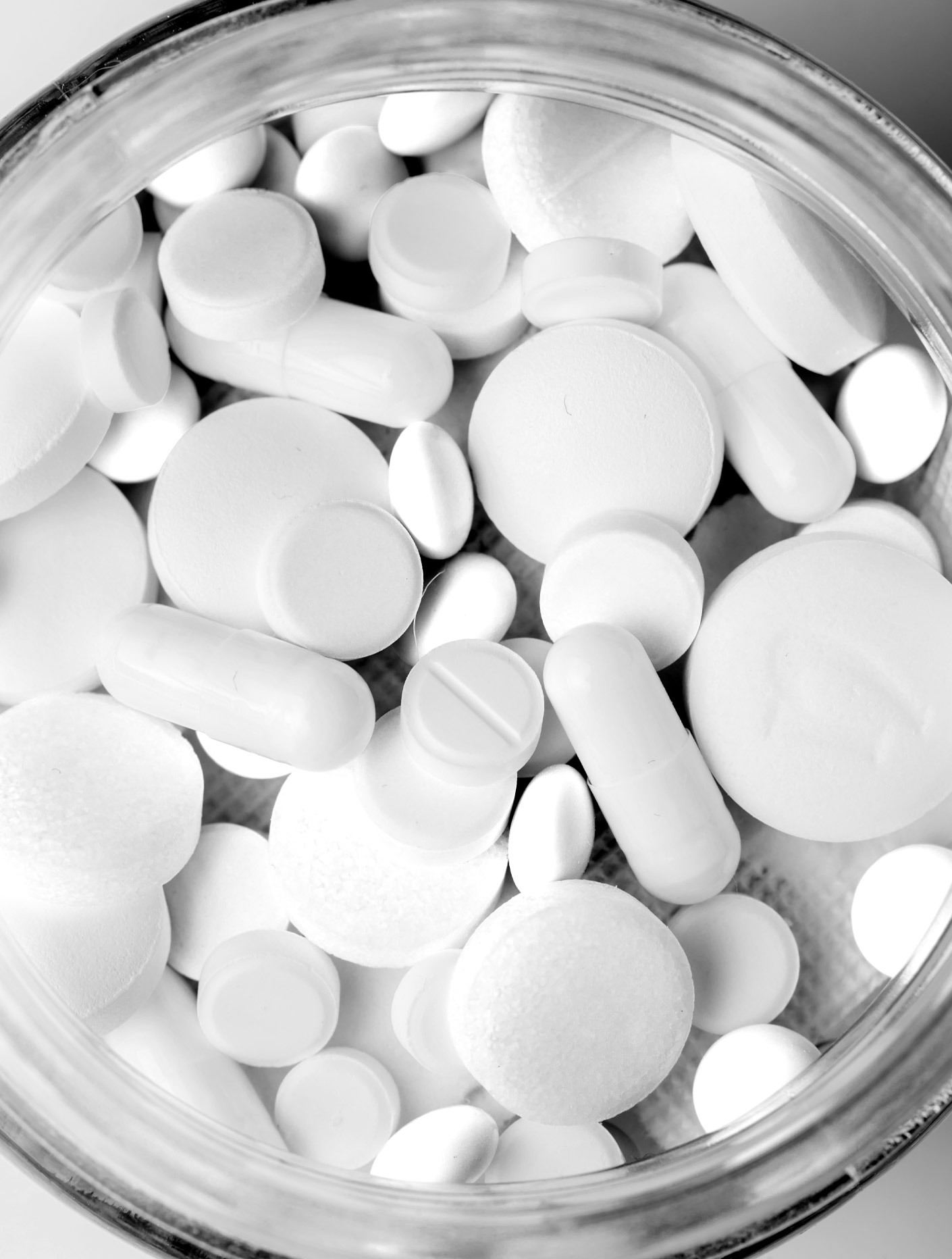
Dangers of drug misinformation
The ability for AI tools to provide guidance on medications, including drug interactions, was called into question by researchers from Long Island University (LLU), who presented their study findings at the American Society of Health-System Pharmacists (ASHP) Midyear Clinical Meeting Dec. 3-7, 2023, in Anaheim, California.
They challenged ChatGPT with real questions posed to the LLU College of Pharmacy drug information service over a 16-month period in 2022 and 2023. Only 10 of the 39 ChatGPT provided responses were judged by pharmacists to be satisfactory, while responses to the other 29 questions did not directly address the question, were inaccurate, and/ or incomplete.
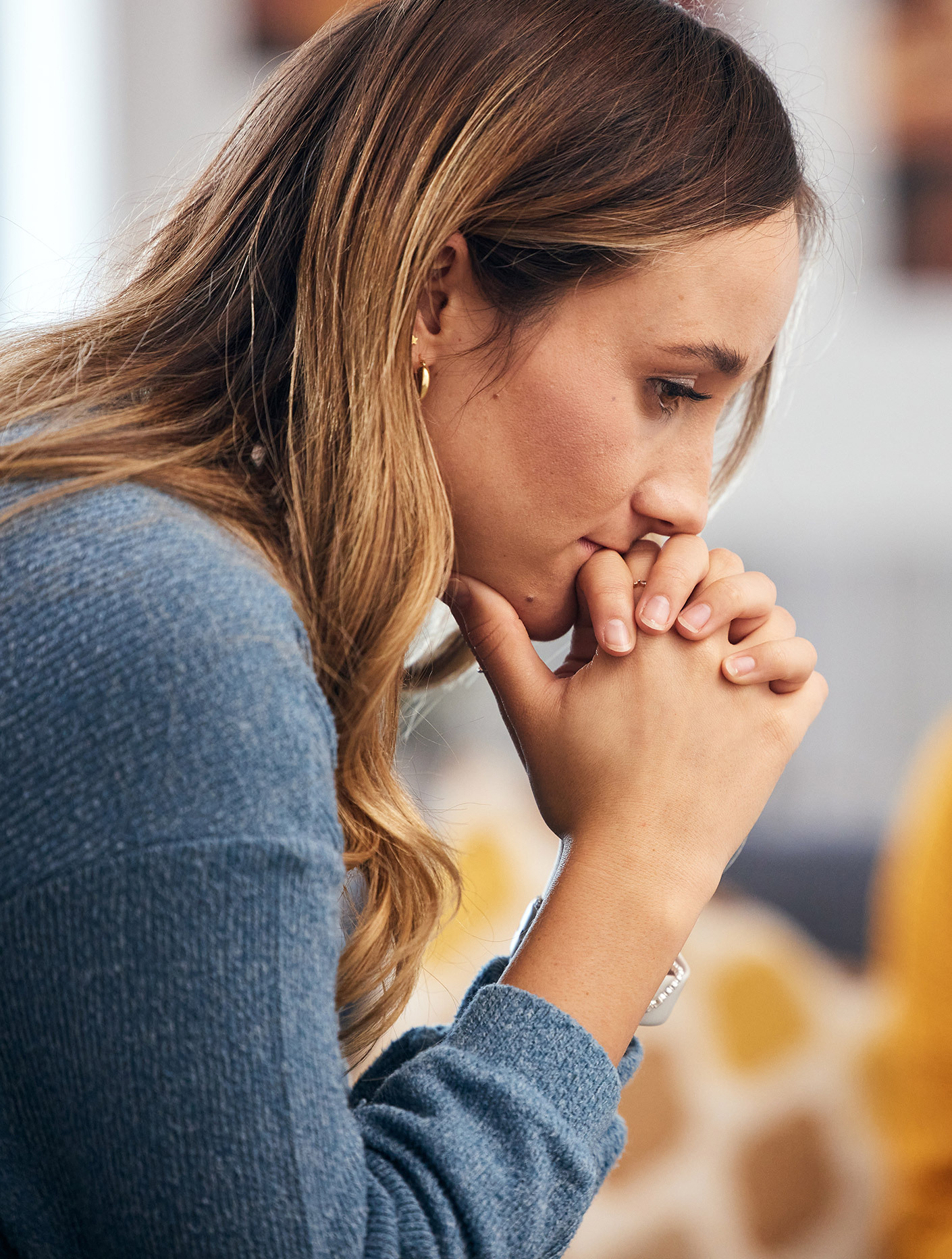
Ethical issues in behavioral health
The risks of using AI to diagnose and treat patients were also evidenced in recent research studying ethical issues with using chatbots in mental health, published in the 2023 edition of the Digital Health journal. The researchers noted how current mental health chatbots:
- Cannot grasp the nuances of social, psychological and biological factors that feed into mental health difficulties
- Cannot fully replicate the range of skills and the affective dimensions of a human therapist or entirely replace the practitioner
- May potentially cause harm to some people and thus not align with the principle of non-maleficence
Why data accuracy and completeness matters
One of the greatest challenges in leveraging AI and ML today in healthcare is inaccurate and incomplete data.
“The lifeblood of AI/ML is data,” stated John League, CFA, Head of Digital Health Research for Advisory Board in a recent NEJM Catalyst article. “The reality of health care data is that it is often most accurately described as ‘messy.’”
AI algorithms trained on poor quality data present risks to the patient in terms of false diagnosis and treatment recommendations, coupled with biases that reflect societal inequities.
“Even fractional amounts of poor-quality data can substantially hamper AI performance” - Scientific Reports
“The accuracy of AI models is only as good as the data they are trained on, and if the data is biased or incomplete, it could lead to inaccurate results,” said pediatric radiology fellow Som Biswas, MD in his Georgetown Journal of International Affairs article. “In medicine, inaccurate diagnoses or treatment recommendations can have severe consequences, including harm to patients or even death.”
According to Biwas, the data for AI training must be comprehensive, unbiased, and of high quality, adding how diverse data sets from a variety of demographic groups can help reduce bias in AI models.
Complete and accurate data collection via chat-based care
Speed to a doctor is essential to the quality and effectiveness of our healthcare; however, the growing scarcity of primary care physicians in the U.S. has increased the distance between patient and provider.
To alleviate the burden on physicians and provide high quality patient support with efficiency and convenience, CirrusMD has developed its Physician-first Care & Guidance model. Through it, patients are connected to physicians in less than a minute from the time they decide to take action, 24 hours per day, 365 days per year via chat-based text exchange.
CirrusMD’s board certified physicians support each patient uniquely and holistically. They have access to each patient’s health information, health plan and benefits, and in-network resources. They can deliver primary, urgent/acute, chronic, and/or preventative care services immediately and longitudinally where appropriate, depending on what the patient needs during the care encounter.
The chat-based text exchange between the physician and patient is stored verbatim in their natural language. If either party failed to document any detail of their encounter, they can refer to the chat text to review what was discussed.
Patient and physician can connect again within a 7-day window to address additional care needs, gaps or concerns that may arise. If a patient has a follow up question about their care, forgot to mention something during the original encounter, or their condition has changed and they need additional support, the physician is only a chat away.
To date, CirrusMD has captured more than 50M one-on-one chat text exchanges between physicians and their patients. Because the care episodes are text based and document the exact words communicated, these records are a 100% accurate representation of the encounters.
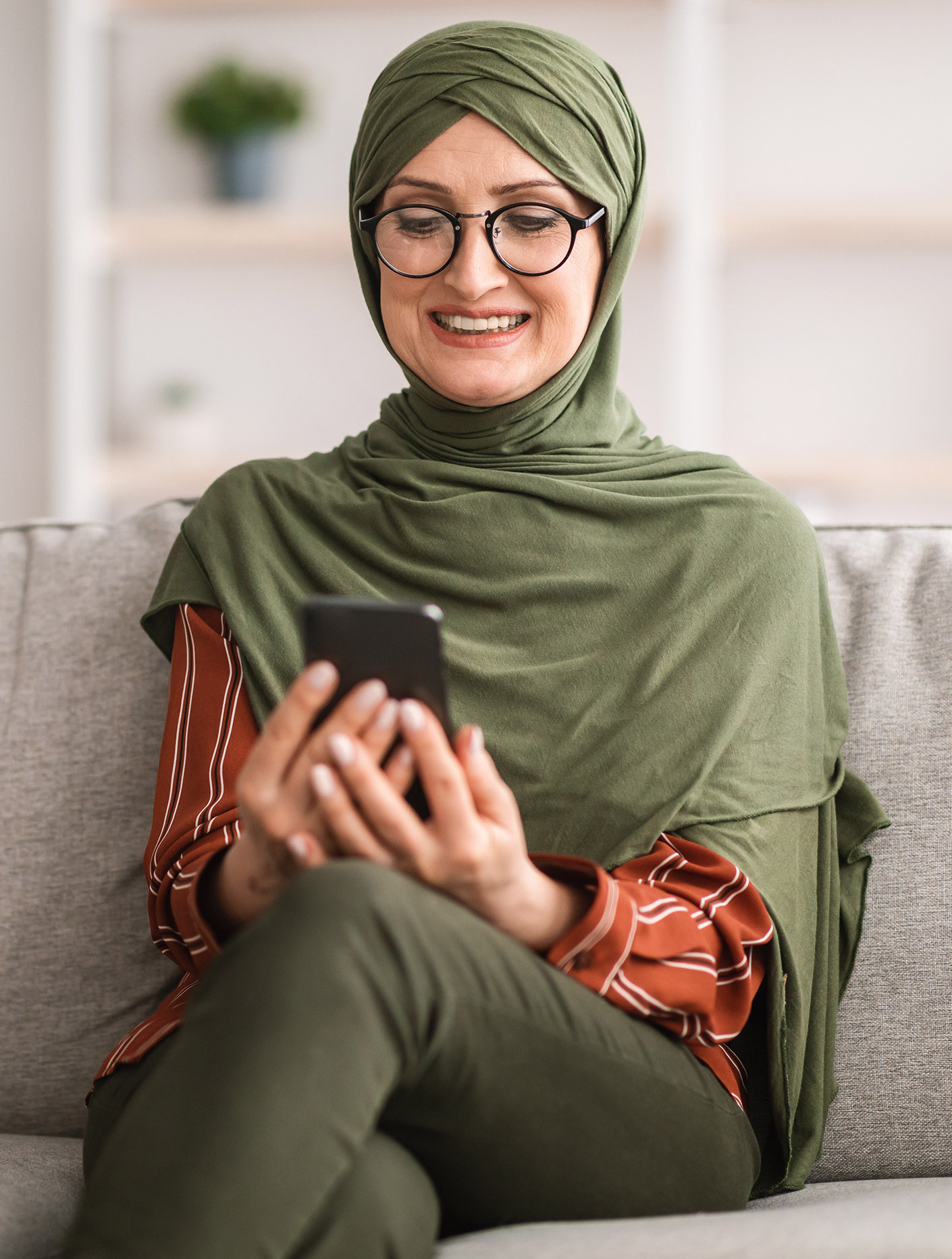
UCF NSF-Funded study on chat-based data completeness
The University of Central Florida (UCF) and CirrusMD partnered on a National Science Foundation (NSF) funded research project to characterize the data completeness of chat-based episodes of care on the CirrusMD Physician-first Care & Guidance virtual care platform. They also tested the hypothesis that high data completeness correlates positively with patient experience and outcomes. The UCF research team analyzed a deidentified data set from CirrusMD’s physician/patient one-onone chat text exchanges - 84 unique conversations, with a total of 6,259 sentences or lines - for data completeness and physician/patient sentiment.
They found CirrusMD chat exchanges to have:
- Excellent data completeness
- Thorough physician documentation
- Clear communication between physician/ patient
Employing AI and ML to support the physician-first care
CirrusMD is now leveraging its vast quantity of accurate and complete data for AI/ML enabled intelligence, where the technology doesn’t replace the physician or come between the physician and patient, but instead, surfaces valuable insights for physicians to consider during patient chat encounters.
The CirrusMD physician and technology teams are closely collaborating on these advancements – combining clinical and technical knowledge to deliver safe, effective solutions. Working together they are putting the power of AI and ML behind physicians while the physicians continue to apply their training, skills, experience, and expertise to engage directly with the patients and guide their care pathways.
The goal is to empower the physician with real-time information at their fingertips during an episode of care, such as resources related to the patient’s condition, rather than the physician having to direct their attention away from the patient to search for this information. The physician evaluates the information surfaced and uses their individual knowledge, experience, and expertise to decide whether to employ it.
Regarding protected health information (PHI) and data security, the company is applying AI and ML to general clinical condition and resource data, and not to patients’ personal details.
Surfacing insights and resources
The CirrusMD technical team has developed the Clinical Intelligence Engine (CIE), which proactively “listens” to identify patient needs and create real-time recommendations against medical categories, including behavioral health, dental, muscular-skeletal (MSK) conditions, and hypertension.
A team of CirrusMD physicians review physician/patient chat-based text exchanges (their own and those from other physician/patient encounters). They use a proprietary clinical annotation tool to identify and notate information in encounters related to specific care needs and/or resources.
For example, a physician may review chat messages between a CirrusMD physician and patient regarding the patient’s primary complaint, knee pain, but during the encounter the patient also mentioned they were anxious and losing sleep. This could be an indication of behavioral health issues, such as anxiety and/or depression.
Using the clinical annotation tool, the physician reviewing the encounter notes what resources they would like to have available on demand when a patient presents in a similar way. Perhaps it is access to GAD 7 and PHQ9 anxiety and depression screening tools that the patient can complete during the encounter so the doctor can assess the results and make recommendations based on them.
The CirrusMD technical team then trains its CIE on the clinical annotation data so it can continuously “listen” to encounters, identify potential patient needs beyond the primary complaint, and make real time recommendations. This allows the physician to consider these recommendations, and combine these insights with their own expertise and judgment, then utilize available resources during an encounter without having to navigate away from the conversation, all in real time.
When the CIE delivers an inference and suggests resources to the physician during a patient encounter, the physician can click a thumbs up or thumbs down to accept or reject the resources, essentially informing the CIE as to whether this was an accurate prediction. In this way, the model continuously learns and improves its accuracy based on physician feedback.
Healthcare benefit-specific resources
Navigating health insurance is a burden on patients, with research showing that many adults lack knowledge of their covered benefits. A 2023 KFF survey of consumer experiences with health insurance revealed that (51%) of insured adults find at least one aspect of how their insurance works at least somewhat difficult to understand.
Health benefit nuances also burden physicians with timeconsuming administrative work. In a conventional physician/ patient care encounter, in-person or virtual, the physician wanting to refer a patient to a resource would have to research whether it was covered within that patient’s insurance package, in turn taking physician’s time away from the patient during a care encounter.
To make the surfaced resources even more relevant and valuable to physicians and patients, the CirrusMD team is training the CIE to match against resources specific to that patient’s specific employee benefits package. The company refers to this model as a Dynamic Playbook.
For instance, a physician and patient are chatting, and the patient mentions they are struggling to lose weight, which is impacting their mobility. The CIE detects this signal and finds the patient resources relevant to these types of patient conditions (e.g. weight loss programs, physical therapy), filters them to only those resources covered by the employee’s individual insurance plan, and presents them to the physician in real-time during the encounter. Again, the physician determines whether it makes sense to share this information with the patient.
Immediate access to relevant resources and covered benefits also helps close the loop on referrals and follow up care. In a current pilot program of this smart technology solution with a large U.S. employer, there was a 400% increase in referrals for behavioral health resources to patients. This contrasts sharply with typical rates of completion for diagnostic tests and referrals, which are low for all patient/physician visit types but even worse when ordered during telehealth visits.
Coding and billing efficiency
ICD-10-CM (International Classification of Diseases, Tenth Revision, Clinical Modification) is one of the primary medical coding classifications physicians use to bill for patient services provided. With around 68,000 ICD-10-CM diagnosis codes, identifying and recording the correct codes is a major administrative burden on physicians. Incorrect coding or failure to code for a service can result in lost revenue, even charges of fraud or abuse.
Researchers have been exploring the use of AI and ML to automate coding of patient encounters for greater accuracy and to relieve physicians of the burden. In a 2023 article published in the NPJ Digital Medicine journal, Harvard Medical School researchers stated:
“Artificial intelligence (AI) and natural language processing (NLP) have found a highly promising application in automated clinical coding (ACC), an innovation that will have profound impacts on the clinical coding industry, billing and revenue management, and potentially clinical care itself.”
CirrusMD has made tremendous progress in this area, training its CIE to review all progress notes written by the company’s physicians during patient encounters to identify the ICD-10- CM codes used. Now, as a doctor is writing a progress note, this Clinical Diagnosis Assistant suggests codes based on an analysis of the progress note text in real-time.
As with all the company’s other AI/ML applications, the power is in the hands of the physician. If the physician agrees with the codes presented by the CIE, they can skip the step of researching them. If not, they can search out the appropriate codes on their own using the CirrusMD platform’s search function.
With this model in place, CirrusMD physicians are using the platform’s search function 80% less. In other words, 80% of the time the codes presented by the CIE are being accepted by the physicians. This correlates to a decrease in physician administrative time of approximately 2.5 minutes per each patient chat – each chat is typically about 15 minutes in duration.
Conclusion
The surge of interest and apprehension surrounding the integration of artificial intelligence (AI) and machine learning (ML) in healthcare necessitates a nuanced approach. Striking a balance is imperative to address concerns and capitalize on the potential benefits offered by AI/ML.
Rather than displacing or creating barriers in physician-patient interactions, the most advantageous strategy involves deploying AI/ML to support physicians during episodes of care. The CirrusMD Physician-first Care & Guidance virtual care model positions the analytical power of AI/ML behind the physician so the personal connection between physicians and patients is preserved, while enhancing the efficiency of care interactions.
This collaborative approach not only facilitates better-informed decision-making, but also has the potential to deliver higher quality care to a larger patient population at a reduced cost, showcasing the transformative impact of thoughtfully integrating AI/ML into healthcare practices.
References
1. 60% of Americans Would Be Uncomfortable With Provider Relying on AI in Their Own Health Care, Pew Research, February 22, 2023, https://www.pewresearch.org/science/2023/02/22/60-of-americans-would-be-uncomfortable-with-provider-relying-on-ai-in-their-own-health-care/
2. Medscape Physicians and AI Report 2023: A Source of Help or Concern? Medscape, October 30, 2023, https://www.medscape.com/slideshow/2023-artificial-intelligence-6016743#7
3. Augmented Intelligence Development, Deployment, and Use, American Medical Association (AMA), November 14, 2023, https://www.ama-assn.org/system/files/ama-aiprinciples.pdf
4. Health is Primary, Primary Care Collaborative’s (PCC) 2023 Evidence Report, November 2023, https://thepcc.org/sites/default/files/resources/pcc-evidence-report-2023.pdf
5. The shrinking number of primary-care physicians is reaching a tipping point, The Washington Post, September 5, 2023, https://www.washingtonpost.com/opinions/2023/09/05/lack-primary-care-tipping-point/
6. Health is Primary, Primary Care Collaborative’s (PCC) 2023 Evidence Report, November 2023, https://thepcc.org/sites/default/files/resources/pcc-evidence-report-2023.pdf
7. AMA Augmented Intelligence Research, American Medical Association (AMA), November 2023, https://www.ama-assn.org/system/files/physician-ai-sentiment-report.pdf
8. Basit, Abdul. (2023). Enhancing Healthcare Delivery through the Integration of Artificial Intelligence and Machine Learning: A Comprehensive Analysis, https://www.researchgate.net/publication/375810153_Enhancing_Healthcare_Delivery_through_the_Integration_of_Artificial_Intelligence_and_Machine_Learning_A_Comprehensive_Analysis
9. Can Artificial Intelligence–Driven Chatbots Correctly Answer Questions about Cancer? National Cancer Institute (NCI), October 3, 2023, https://www.cancer.gov/news-events/cancer-currents-blog/2023/chatbots-answer-cancer-questions
10. Chen S, Kann BH, Foote MB, et al. Use of Artificial Intelligence Chatbots for Cancer Treatment Information. JAMA Oncol. 2023;9(10):1459–1462. doi:10.1001/jamaoncol.2023.2954
11. Generative AI for Health Information: A Guide to Safe Use, Yale Medicine, January 8, 2024, https://www.yalemedicine.org/news/generative-ai-artificial-intelligence-for-health-info
12. Study Finds ChatGPT Provides Inaccurate Responses to Drug Questions, ASHP News Center, December 5, 2023, https://news.ashp.org/News/ashp-news/2023/12/05/study-finds-chatgpt-provides-inaccurate-responses-to-drug-questions
13. Coghlan S, Leins K, Sheldrick S, Cheong M, Gooding P, D’Alfonso S. To chat or bot to chat: Ethical issues with using chatbots in mental health. Digit Health. 2023 Jun 22;9:20552076231183542. doi: 10.1177/20552076231183542. PMID: 37377565; PMCID: PMC10291862. https://pubmed.ncbi.nlm.nih.gov/37377565/
14. Confronting the Reality of AI/ML in Care Delivery, NEJM Catalyst, March 16, 2022, https://catalyst.nejm.org/doi/full/10.1056/CAT.22.0072
15. Dakka, M.A., Nguyen, T.V., Hall, J.M.M. et al. Automated detection of poor-quality data: case studies in healthcare. Sci Rep 11, 18005 (2021). https://doi.org/10.1038/s41598-021-97341-0
16. Revolutionizing Healthcare: The Promises and Pitfalls of AI in Medicine with ChatGPT, Georgetown Journal of International Affairs, June 28, 2023, https://gjia.georgetown.edu/2023/06/28/revolutionizing-healthcare-the-promises-and-pitfalls-of-ai-in-medicine-with-chatgpt/
17. AI Adoption in U.S. Health Care Won’t Be Easy, Harvard Business Review, September 14, 2023, https://hbr.org/2023/09/ai-adoption-in-u-s-health-care-wont-be-easy
18. Gurupur, V., Shelleh, M., Leone, C., Schupp, D., Azevedo, R., Dubey, S. (2023). THNN - A Neural Network Model for Telehealth Data Incompleteness Prediction, Proceedings of the 44th Annual International Conference of the IEEE Engineering in Medicine and Biology Society (EMBC’23), July 24 – 28, 2023, Sydney, Australia. In Press
19. Zhong A, Amat MJ, Anderson TS, et al. Completion of Recommended Tests and Referrals in Telehealth vs In-Person Visits. JAMA Netw Open. 2023;6(11):e2343417. doi:10.1001/jamanetworkopen.2023.43417. https://jamanetwork.com/journals/jamanetworkopen/fullarticle/2811870
20. Venkatesh, K.P., Raza, M.M. & Kvedar, J.C. Automating the overburdened clinical coding system: challenges and next steps. npj Digit. Med. 6, 16 (2023), https://pubmed.ncbi.nlm.nih.gov/36737496/