Video Transcript
Tanya Dillard. Senior Vice President, Product Management & Innovation, CirrusMD (00:00):
We'll probably maybe a little bit tired, maybe a little bit more hoarse than how we started the conference initially, but we really appreciate you sticking around for this particular talk. I am Tanya Dillard. I am the head of product management and innovation for CirrusMD.
Zayed Yasin, MD. Healthcare and Life Science team, Writer (00:15):
I'm Zayed Yasin. I'm the healthcare industry lead for Writer and also a practicing emergency physician is my side hustle.
Tanya Dillard. Senior Vice President, Product Management & Innovation, CirrusMD (00:26):
Alright, so who is CirrusMD? So we are a chat first virtual primary care platform. We are, we deliver care across the United States in all 50 states. We have physicians that are licensed in all 50 states and we meet our patients in under 60 seconds, not with a chatbot, not with a triage tool. Every time a patient comes onto our platform, they need a true doctor in less than 60 seconds. In fact, we are at about 15 seconds in time to connect with our patients. We're headquartered in Denver, we've been around for 12 years. Patients love us. We are able to solve their issues and about 83% of the time virtually on the platform without any additional work, if you come once, you're more likely to come back. 47% of the people on our platform are return users and we're really proud of our 96% satisfaction score from our patients. So our patients love us, our buyers love us. We have 13 million patients now on our platform and we have been able to deliver a little over a billion dollars of savings across our direct to employer clients as well as our government and as well as our payer clients. And we recently just published a study with an actuarial firm that was proving our three to one guarantee, ROI. So that's sru.
Zayed Yasin, MD. Healthcare and Life Science team, Writer (01:46):
And so we're Writer. We're a full stack for full stack enterprise generative AI platform and we are the way to get generative AI into all parts of your company to deliver impact responsibly at scale quickly. We have a little bit more about how we do that as we get into the presentation, but I think what's worth knowing is that we've been around since 2020, which is the short time as companies go by generative AI a long time. So we've had the chance to understand how do you really use this technology and get it into actual impact, which is why 40 of the Fortune 500 companies use us to deliver their generative AI solutions.
Tanya Dillard. Senior Vice President, Product Management & Innovation, CirrusMD (02:26):
Okay, so what brings CirrusMD and together? So to understand that you have to understand the business problems that CirrusMD is trying to solve and that really starts with how we think about the point of care. We believe that the point of care is really one of the most impactful points on a journey in healthcare to affect positive outcomes, whether that's clinical outcomes, financial outcomes, or even patient satisfaction. So with that, we think about alright, how do we make the point of care more effective? It's a really special time where you have a patient who has a need and that means they are activated, they are open, they are ready to listen, they are motivated to actively information that a physician gives them. And a physician is really unique in this space. They're the only ones who can do four things. They're the only ones who can triage, diagnose, treat, and refer the only ones who can do all that.
So you have this very unique space in the care journey, but what we find is that there's really critical information that's missing out in that care journey. And so we started to contemplate what kind of great information could we get in at the point of care that is helpful to the physician to have a really effective care plan for those better outcomes. And so as we thought about that type of information that you could get in, whether it's additional benefits information, whether it's additional patient information, how could we do that effectively? That's not going to increase the burden of the physician. Physicians are very protective of their workflows, rightfully so. It has to be seamless. So as we started to contemplate that, we started talking to our ML engineer internally and started to see or ask the question, could we use AI to help with this problem of getting good information in?
And we were in agreement, yes, AI has great promise for this, so let's start to develop criteria that supports our business and responsibly uses ai. And so here's what we came up with. If we were going to go down this path, it had to bring in quality information that enriches the experience, enriches the encounter. It cannot be noise, it must be signal. It also had to still uphold physician satisfaction. We are all about trying to give a new proposition to physicians who practicing medicine. So it wasn't going to work if they had more administrative burden. It needed to reduce that and it needed to do it in a way that they would radically adopt this technology. It also needed to support our speed to contact with our patients. It was never on the table for us to use AI in a way that comes between our patients and our providers.
We were never going to use it as a chat bot. We always had to use it with the speed of contact in mind that needed to be under 60 seconds. It also needed to scale with us. We knew that at some point we would start with nonclinical use cases, but then we would up the ante and start to use more clinical use cases. So it needed to be able to scale across both of those. And then of course cost of course is always a concern, but it also needed to help us maintain affordability but without rationing care. And that's often how AI I think is employed with a lot of companies is they're starting to think about how do I use it as a care rationing mechanism rather than a care enhancement mechanism. So we've got our criteria, we're going to solve these problems often to the world.
We go with our single ML engineer, we are going to build it all. But as we started to experiment, we were working with open AI's GPT models and they were rapidly iterating on their models. We had GPT-3, 3.5, 3.5, turbo four point, and as they were iterating every time it was kind of hitting us back to square one with our product development. And so we really were having unpredictable performance and it was causing us to push our product to launch. Its out. We also, again were kind of concerned that the GPT models were not medically, they're not based on medical information, so they are trained on all of the internet and that's fine for non-medical use cases. But as we started to contemplate medical use cases, we wanted a generative a I model that could understand medical jargon, medical terminology and that's not something that the open AI models were going to be able to handle probably very well.
We weren't super confident about that. And then finally we have our one ML model or our one ML engineer who's going to do all of this wrong. As we continue to build, we continue to learn and we continue to realize we were really under-resourced for this endeavor. We needed to add more people, add more infrastructure. And as the scope of this project continued to grow, we had to say stop. Hold on. Are we an AI company or are we a healthcare delivery company? We're a healthcare delivery company empowered by technology. It is not our north star to be an AI company. We want to solve access problems in primary care. And so we stopped the internal build and we commenced an external search and that is what brought us to Writer.
Zayed Yasin, MD. Healthcare and Life Science team, Writer (07:47):
I think what the story that you heard from Tanya about sirius's journey is really the natural history for enterprise generative ai. It's a story that we've seen in different variants with almost all of the companies that we work with. Generative AI is obviously transformative and has incredible potential. Let's start building it themselves until you realize it's hard doing this really well with the level of reliability, responsibility, deployability scale is not easy and so most companies large and small, they go through this process, they realize we can't do this ourselves. Building it with engineers, it takes a really long time. It's really, really expensive. And unless you are a truly AI company, you'll never have the number of engineers and technology that you have in order to do everything the business needs. I think one of the learnings from generative AI is that there is no one killer app, not just organization, but every function has 5, 10, 20 different things that need to be generative AI enabled and your internal tech team will never be able to build them all.
And if you want to buy all from different point solutions, it becomes unscalable and ungovernable. You can't treat be like if you treated this environment and as shuffle as a bizarre everybody against my carrot sea, some money needs here, some brown people over there and put it all together. That doesn't work for the way you need to get generative ai. And so the way we solve this is to the next slide. By having a unique full stack approach, we have all the pieces that are built to work together, functioning like building with Legos, not sculpting raw metal, which means that we can do this together in a fraction of the time of doing it yourself or working with an agency and engineering type of model where everything has to be built and manually connected. We have a proprietary set of large language models trained on curated data including Paul Meyer me, we'll talk more about that in a moment as well as knowledge graph.
Our way of bringing in through rag the proprietary information that exists in every company and which is a fundamental component to having applications that really work for your use case and in your company and the guardrails to keep it safe, a way of building through our AI studio and integrations so that the building connection goes really fast with the kind of customization that you'd otherwise need engineers for. And because we're doing it for so many companies, we've seen this show go on so many times. We do AI program and change management so that you can learn from the best practices in the industry. When we walking through the process and a little bit more about Meyer Med to what Tani was saying before, medicine is his own language. Our models all speak 33 different languages, but some of them also speak medicine, which really is its own language and having a very high performing model has been important for our medical applications. And all minor med is by a substantial margin, the highest performing medical specific large language model that is available to anyone today.
Tanya Dillard. Senior Vice President, Product Management & Innovation, CirrusMD (11:05):
Yeah, this when we were really looking through the vendors, this really is one of the differentiators for us that we would be able to scale with a large language model that really did understand the language of this. Okay, so let's talk about the use cases. So pulling in good information into the point of care. So the first one was benefits navigation at the point of care. So our hypothesis is that if a provider recommends a third party resource, so I'll talk about what that means, opponent a patient is much more likely to use it because it's recommended by their physician and it's in their care plan. Now these third party resources, most physicians don't know that they exist. Many of these third party resources bought directly by employers. Many of these companies are actually exhibiting here today, the Sword Health, the Omada of the world, but physicians have no idea that they exist and that they're available to a patient and they may actually work really well inside the care plan that has already been defined.
And so we thought can we bring that into the physician workflow so that the physician could if you would consider prescribe it as something that's part of their care plan. And so we took that on. We had built this or started down this path, building it manually first and then we kind of had to stop the build and we started working with Writer on this. So if you can think of it this way, we constructed in such a way where the AI intelligence reads what's in the chat and can understand exactly what the chat is about. For example, somebody shows up with a behavioral health need, we screen them, perhaps they're appropriate for A-S-S-R-I prescription. So the chat says, oh, this is a behavioral health, or the AI says this is a behavioral health chat rate. I'm now going to go out and find any relevant benefits and bring them, return them back to the doctor into their workflow in our EMR.
The doctor clicks on the button says, oh, this person also has therapy free therapy available through let's say spring health available through spring health. That also might be something that they would be interested in not just taking the medication. I'm going to give this to them in their care plan. We were really pleased with the results of this and so over our manual, because we were doing this manually first compared to the manual usage, our providers were using it 400% more. That means they were looking at it, they were recommending it to patients 234% more. There's a lot of reasons. There's a delta between the two that are clinical in nature. It's not always appropriate to refer sometimes to these third party benefits. We give our providers the ability to make that decision. Again, we're not going to put AI in front of our patients.
It is really a tool for our providers. They then make the determination of whether or not they're going to share this. The cool thing about this is it validated our hypothesis. Yes, if a provider made the recommendation to use that particular benefit and put it in the care plan, we actually saw a 30% increase in the patients engaging in those resources. So we were thrilled with that. Additionally, we were able to get this use case off the ground in less than four months. It was actually closer to about two months. So we were really thrilled with the results there. Second use case. So this is where we start to say, great, we're now going to put more information into the point of care for providers. How do we start to reduce provider burden? And so we started to think through how could we start taking the documentation burden off of our providers?
Our providers, like all providers are really interested in patient care. When it gets busy on the platform, you might imagine that our providers kind of defer the documentation. We also know that's not great practice. So how could we in the moment, again, survey the chat, we understand or the AI understands that this is a musculoskeletal chat, chat, summarize the chat, return a summary to the physician, they can then say, yes, this is correct, or edit it if need be and then move on with their day. Their documentation is done. What you see in front of you is actually screenshot of our app and the back and forth between a patient and to the right, you see just kind of the summarized soap note if you will, that would be presented to the provider to approve or we're excited about this. This is being in test right now.
We predict that this is going to be about a 20% time saver for documentation for our providers. So they're very excited for us to get automated progress needs off the ground. Then they can see more patients, which is what they love to do. So I think it's important to kind of take a step back in contrast kind of the internal build. So we had 12 months, at which point we still didn't have a viable product. Compare that to the six months, which not only were we able to get our first use case off the ground, but we're in testing for our second use case. So we've been really pleased with the progress that we've been made. With runner. I would say there's a few things that I would want to leave you with at the end of this talk is generative AI has incredible potential.
We see it everywhere, but I would say be pragmatic and start with concrete value use cases first. Also start with lower risk use cases first nonclinical, and then step into the more, a little bit higher risk use cases with the clinical side of it. And then if there's nothing you can remember from what I said today, it should be Elise's. One thing, doing it on your own is hard and it's slow. If it is not your north star, as it was not ours, go and invest in the expertise of those companies who do this. We were super happy with the decision that we made and it has allowed us to now really focus on what we do, which is 20 care.
Zayed Yasin, MD. Healthcare and Life Science team, Writer (17:09):
Thank you Tanya. And I think I want to echo that we think that generative ai, everyone should be using it. Almost no one should be building it themselves unless that is really what you do day in and day out because it requires a certain technical basis that you can't do as a side project or at a SCWorks inside a company whose real strength is somewhere else. I love what Tanya said at the end about start, about how do you get started, areas where you can drive value, but where the risk profile is low. So if you look at where we got started together, we started with benefits navigation. It allowed us to learn about how do you use non-deterministic algorithms with data from multiple sources and get that useful into the position of workflow at the point of care. But in an area where if there are hiccups, you can't hurt anybody, you can't break the law, nothing really bad can happen if it doesn't go well as you are learning how to use it.
From there we moved on to clinical documentation, understanding what is clinical language, like how is it processed in serious' context? So now we understand non-deterministic algorithms, we understand point of care decision and support, we understand clinical language and now we are the process talking about what are the next steps? How do we step up the value chain, go to things that have more complexity, more potential for impact that we wouldn't have wanted to start at, but now we're getting ready. So talking about areas like quality review, no one likes doing chart review. It's tedious. It takes a long time. You can never do more than a fraction of the cases. And it requires your medical directors being scarce system group of people within the company. With ai, we can review every single chart and then only flag for review by the medical directors areas where you really do need that human interaction and have much deeper understanding what's going on clinically. And then once we've understood quality from that point of view, figuring out how do we improve that quality with clinical decision support at the point of care which integrates the learnings from all of those three earlier steps, but where we wouldn't have wanted to get started. So thank you so much for staying around. If you're still here at Health on Wednesday morning, we know you stayed just before this session, so thank you and if you have any questions, we'd love to talk to you some more. Thank you all.
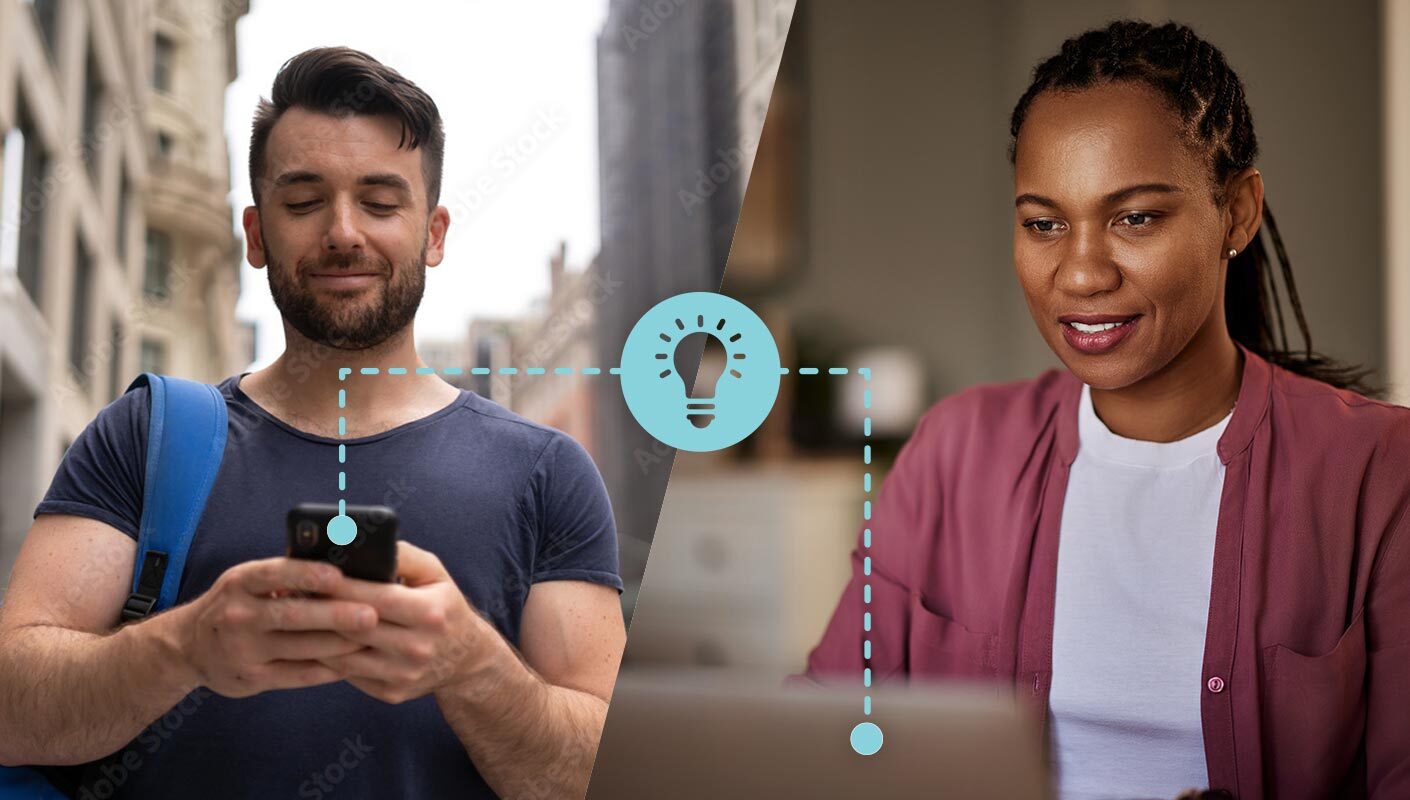
AI in Healthcare White Paper
Earlier this year CirrusMD published a whitepaper, Empowering Physicians and Streamlining Patient Care Interactions with AI/ML. The whitepaper delves into how CirrusMD is leveraging its vast quantity of accurate and complete data for AI/ML enabled intelligence. We believe that AI technology shouldn’t replace the physician or come between the physician and patient, but instead, AI should surface valuable insights for physicians to consider during patient chat encounters. CirrusMD understands how AI and ML can be used as highly effective tools to accelerate physician workflows and deliver more customized care to their patients — and not to replace an actual doctor.